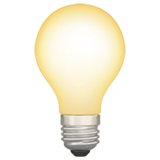
Article Snapshot
Engineering Data Management encompasses the processes, tools, and strategies employed to acquire, store, organize, integrate, analyze, and maintain engineering data throughout its lifecycle. It is a multidimensional discipline that addresses the governance, acquisition, storage, integration, analysis, and maintenance of engineering data, ensuring its quality, accessibility, security, and usability.
The Importance of Engineering Data Management
Effective Engineering Data Management is critical for organizations operating in engineering-intensive industries such as manufacturing, construction, aerospace, automotive, and many others. It serves as the backbone of successful engineering projects, enabling engineers, designers, and other stakeholders to collaborate seamlessly, make informed decisions, and drive innovation.
By implementing robust Engineering Data Management practices, organizations can achieve several key benefits. Firstly, it ensures data integrity and consistency, reducing errors and rework. Secondly, it facilitates the reuse and sharing of data, promoting collaboration and eliminating silos. Furthermore, it enhances data security and compliance, protecting valuable intellectual property and sensitive information. Lastly, Engineering Data Management enables organizations to gain valuable insights from data analysis, optimizing processes, improving product quality, and driving continuous improvement.
The Engineering Data Management Process
The Engineering Data Management process comprises several interconnected stages, each contributing to the overall efficiency and effectiveness of data management. It begins with data governance and policy, where organizations establish frameworks, policies, and standards to govern data management activities and ensure compliance with regulatory requirements.
The next stage involves data acquisition and capture, where engineering data is sourced from various internal and external sources. This includes data from design and simulation tools, sensor networks, test equipment, supplier databases, and more. Data quality and validation techniques are applied to ensure the accuracy, completeness, and reliability of the acquired data.
Once the data is collected, it needs to be stored and organized effectively. This involves selecting appropriate storage systems such as databases or cloud storage solutions. Additionally, data classification and taxonomy are implemented to categorize and structure the data, enabling easy retrieval and analysis. Data security measures, including access controls and encryption, are also put in place to safeguard sensitive information.
The integration and analysis stage focuses on combining disparate data sources, performing data fusion and aggregation, and applying statistical analysis and data visualization techniques. This enables engineers and decision-makers to gain valuable insights, detect patterns, and make data-driven decisions. It also facilitates collaboration among teams, allowing them to work cohesively toward achieving project goals.
Finally, the data maintenance and lifecycle management stage involves archiving and backing up data, managing data versions and changes, and ensuring compliance with retention and disposal policies. Regular data backups and disaster recovery plans are essential to mitigate the risk of data loss and ensure business continuity.
In the following sections of this blog post, we will delve deeper into the components of Engineering Data Management, explore the tools and technologies available, and discuss best practices for effective implementation. By the end, you will have a comprehensive understanding of what Engineering Data Management entails and how it can revolutionize the way engineering organizations operate. Let's dive in!
Introduction to Engineering Data Management
Engineering Data Management is a crucial discipline that plays a pivotal role in the success of engineering projects and organizations. In this section, we will explore the definition and importance of Engineering Data Management, providing an overview of the process and discussing the key benefits and challenges associated with it.
Definition and Importance of Engineering Data Management
Engineering Data Management can be defined as the systematic and strategic approach to acquiring, organizing, integrating, analyzing, and maintaining engineering data throughout its lifecycle. It encompasses a range of activities and processes that aim to ensure the quality, accessibility, security, and usability of data in engineering projects and organizations.
The importance of Engineering Data Management cannot be understated. In today's digital era, where engineering projects are becoming increasingly complex and data-driven, organizations must effectively manage their engineering data to gain a competitive edge. By implementing robust Engineering Data Management practices, organizations can enhance collaboration, optimize processes, improve product quality, and drive innovation.
Overview of the Engineering Data Management Process
The Engineering Data Management process consists of several interconnected stages that collectively enable organizations to effectively manage their engineering data. These stages include data governance and policy, data acquisition and capture, data storage and organization, data integration and analysis, and data maintenance and lifecycle management.
Data Governance and Policy: This initial stage involves the establishment of a data governance framework and the development of data management policies and standards. It ensures that data management activities are aligned with organizational objectives and regulatory requirements. By defining roles, responsibilities, and processes, organizations can govern their engineering data effectively.
Data Acquisition and Capture: Data acquisition and capture involve sourcing data from various internal and external sources. Engineering data can originate from design and simulation tools, sensor networks, test equipment, supplier databases, and more. It is crucial to employ methods that ensure data quality and validation to guarantee the accuracy and reliability of the acquired data.
Data Storage and Organization: Once the data is acquired, it needs to be stored and organized in a manner that facilitates easy retrieval and analysis. Organizations can utilize various types of data storage systems, such as databases or cloud storage solutions. Additionally, implementing data classification and taxonomy enables efficient categorization and structuring of data, improving accessibility and usability. Data security measures, including access controls and encryption, are also critical to protect sensitive information.
Data Integration and Analysis: The integration and analysis stage involves combining disparate data sources, performing data fusion and aggregation, and applying statistical analysis and data visualization techniques. This enables engineers and decision-makers to gain valuable insights, detect patterns, and make data-driven decisions. Effective integration and analysis of engineering data foster collaboration among teams, enabling them to work cohesively toward achieving project objectives.
Data Maintenance and Lifecycle Management: The final stage of the Engineering Data Management process focuses on data maintenance and lifecycle management. It encompasses activities such as data archiving, backup strategies, data versioning, change management, and compliance with retention and disposal policies. Regular data backups and disaster recovery plans are crucial to mitigate the risk of data loss and ensure business continuity.
Key Benefits and Challenges of Engineering Data Management
Implementing effective Engineering Data Management practices brings forth numerous benefits. Firstly, it ensures data integrity and consistency, reducing errors and rework. By establishing standardized processes and protocols, organizations can enforce data quality control measures, resulting in reliable and accurate data. Secondly, Engineering Data Management facilitates the reuse and sharing of data across projects, departments, and even organizations. This promotes collaboration, eliminates data silos, and improves project efficiency.
Furthermore, Engineering Data Management enhances data security and compliance. By implementing access controls, encryption, and other security measures, organizations can protect valuable intellectual property and sensitive information from unauthorized access and data breaches. Additionally, compliance with data privacy regulations, such as the General Data Protection Regulation (GDPR), ensures that data is handled in a legally and ethically responsible manner.
However, implementing and maintaining effective Engineering Data Management practices also pose challenges. These challenges include managing the sheer volume and diversity of engineering data, ensuring data quality and consistency across multiple sources, integrating data from various systems and tools, and addressing the evolving nature of data formats and technologies. Organizations must be prepared to invest in the necessary resources, technology, and expertise to overcome these challenges and reap the benefits of Engineering Data Management.
In the following sections, we will delve deeper into each component of Engineering Data Management, discuss the tools and technologies available, and explore best practices for effective implementation. By the end of this comprehensive guide, you will have a solid understanding of the concepts, strategies, and benefits associated with Engineering Data Management.
Components of Engineering Data Management
Engineering Data Management encompasses various components that collectively contribute to the effective management of engineering data. In this section, we will explore each component in detail, including data governance and policy, data acquisition and capture, data storage and organization, data integration and analysis, and data maintenance and lifecycle management.
Data Governance and Policy
Data governance and policy form the foundation of Engineering Data Management. It involves establishing a framework that outlines the roles, responsibilities, processes, and standards for managing engineering data within an organization. The data governance framework ensures that data management activities align with the organization's objectives, regulatory requirements, and industry best practices.
The development of data management policies and standards is a critical aspect of data governance. These policies define how data should be collected, stored, accessed, shared, and protected. They establish guidelines for data quality control, data retention and disposal, data security, and compliance with regulations. By implementing robust data governance and policy measures, organizations can ensure consistency, reliability, and security in their engineering data management practices.
Data Acquisition and Capture
Data acquisition and capture involve the sourcing, collection, and validation of engineering data from various internal and external sources. In today's digital age, engineering data can originate from a wide range of sources, such as computer-aided design (CAD) software, simulation tools, IoT sensors, test equipment, supplier databases, and more.
To ensure the accuracy, completeness, and reliability of the acquired data, organizations employ various methods and techniques. Data validation and verification processes are applied to identify and rectify any inconsistencies or errors in the collected data. This ensures that the engineering data used for analysis and decision-making is of high quality and can be trusted.
Organizations must also consider data ownership and intellectual property rights when acquiring data from external sources. Clear agreements and contracts should be in place to define the rights and restrictions associated with the use of such data. By implementing effective data acquisition and capture processes, organizations can gather the necessary data to support their engineering projects and initiatives.
Data Storage and Organization
Once engineering data is acquired, it needs to be stored and organized in a manner that facilitates easy retrieval, analysis, and sharing. Data storage systems, such as databases or cloud storage solutions, are used to store the vast amount of engineering data generated throughout the product lifecycle. These systems provide scalability, security, and accessibility to the stored data.
To ensure efficient storage and retrieval, data classification and taxonomy are implemented. Data classification involves categorizing data based on its characteristics, relevance, and importance. This classification helps in organizing data into logical categories, making it easier to locate and retrieve specific information when needed.
Data taxonomy, on the other hand, refers to the hierarchical structure or classification scheme that defines the relationships between different data elements. By using a well-defined taxonomy, organizations can establish a common language for data organization and improve data consistency and understanding across different projects and departments.
Data security is another crucial aspect of data storage and organization. Access controls, encryption, and other security measures are implemented to protect sensitive engineering data from unauthorized access, manipulation, or theft. This ensures that only authorized individuals or systems can access and modify the data, safeguarding valuable intellectual property and confidential information.
Data Integration and Analysis
Data integration and analysis involve combining and analyzing engineering data from various sources to gain valuable insights and support decision-making. In today's complex engineering projects, data is generated from multiple systems, tools, and departments. Integrating this data provides a holistic view of the project, enabling engineers and decision-makers to make informed decisions based on accurate and up-to-date information.
Data integration techniques, such as Extract, Transform, Load (ETL) processes or data warehousing, are employed to consolidate data from different sources into a unified and consistent format. This allows for seamless data integration and reduces the risk of data inconsistencies or duplications.
Once the data is integrated, analysis techniques are applied to derive meaningful insights. Statistical analysis, data mining, and machine learning algorithms can be utilized to identify patterns, correlations, and trends within the data. Additionally, data visualization tools and techniques help in presenting the analyzed data in a visual format, making it easier to interpret and understand the insights gained.
Data integration and analysis enable organizations to uncover hidden patterns, optimize processes, identify potential risks, and make data-driven decisions. It empowers engineers and decision-makers with the knowledge and insights needed to drive innovation, improve product quality, and achieve project objectives.
Data Maintenance and Lifecycle Management
Data maintenance and lifecycle management involve the ongoing management and governance of engineering data throughout its lifecycle. This encompasses activities such as data archiving, backup strategies, data versioning, change management, and compliance with retention and disposal policies.
Data archiving involves the long-term storage of historical engineering data that may no longer be actively used but still holds value for future reference, regulatory compliance, or intellectual property protection. Archiving ensures that data is preserved and accessible when needed, even after the active use phase of a project or product.
Regular data backups are essential to protect engineering data from accidental loss, system failures, or other unforeseen events. Backup strategies should be implemented to ensure that data is regularly and securely backed up to prevent data loss and facilitate disaster recovery.
Data versioning and change management are crucial in engineering projects where multiple versions of a design or product may exist. Keeping track of data versions and changes helps in maintaining a clear audit trail, ensuring that the history and evolution of data can be traced accurately.
Compliance with retention and disposal policies is also essential. Organizations must adhere to legal, regulatory, and contractual requirements regarding data retention periods and disposal practices. Proper disposal of data ensures that sensitive information is securely destroyed, minimizing the risk of data breaches or unauthorized access.
By effectively managing the maintenance and lifecycle of engineering data, organizations can ensure data integrity, compliance, and accessibility throughout the entire lifecycle of a project or product.
Tools and Technologies for Engineering Data Management
Engineering Data Management relies on a wide range of tools and technologies to effectively acquire, store, organize, integrate, analyze, and maintain engineering data. In this section, we will explore the various tools and technologies available for Engineering Data Management, including data management software, data integration and analytics tools, and cloud computing and big data technologies.
Data Management Software
Data management software plays a crucial role in managing and organizing engineering data. These software solutions provide a centralized platform for storing, accessing, and managing data throughout its lifecycle. Some commonly used data management software includes:
1. Database Management Systems (DBMS): DBMS such as Oracle, SQL Server, MySQL, and PostgreSQL are widely used to store and manage structured data. They provide efficient storage, retrieval, and manipulation of data, ensuring data integrity and security. DBMS also support query languages, allowing users to extract valuable insights from the stored data.
Data Integration and Analytics Tools
Data integration and analytics tools are essential for processing and analyzing engineering data from various sources. These tools enable organizations to integrate disparate data sources, perform complex data transformations, and derive insightful analytics. Some commonly used data integration and analytics tools include:
1. Extract, Transform, Load (ETL) Tools: ETL tools such as Informatica PowerCenter, Microsoft SSIS, and Talend are used to extract data from different sources, transform it into a consistent format, and load it into a target data repository. ETL tools enable organizations to automate the process of data integration, ensuring data consistency and accuracy.
2. Business Intelligence (BI) Tools: BI tools, including Tableau, Power BI, and QlikView, provide powerful visualizations and analytics capabilities. These tools allow organizations to explore and analyze engineering data through interactive dashboards, reports, and charts. BI tools enable users to uncover patterns, trends, and insights from engineering data, enabling data-driven decision-making.
3. Data Visualization Software: Data visualization software, such as D3.js, Plotly, and Tableau, focuses on presenting data in a visual format. These tools enable organizations to create interactive and visually appealing charts, graphs, and maps to communicate complex engineering data effectively. Data visualization software enhances data comprehension and facilitates better communication among stakeholders.
Cloud Computing and Big Data Technologies
Cloud computing and big data technologies have revolutionized the way organizations manage and process large volumes of engineering data. These technologies offer scalability, flexibility, and cost-effectiveness, allowing organizations to handle massive amounts of data efficiently. Some key cloud computing and big data technologies relevant to Engineering Data Management include:
1. Cloud Storage and Computing Platforms: Cloud storage platforms such as Amazon S3, Microsoft Azure Blob Storage, and Google Cloud Storage provide scalable and secure storage for engineering data. Cloud computing platforms like Amazon Web Services (AWS), Microsoft Azure, and Google Cloud Platform offer powerful computing resources for processing and analyzing engineering data in the cloud.
2. Hadoop and Spark for Big Data Processing: Hadoop and Spark are popular open-source frameworks used for processing and analyzing big data. These frameworks enable organizations to distribute data processing tasks across multiple nodes, allowing for parallel processing and efficient handling of large datasets. Hadoop's distributed file system (HDFS) provides fault-tolerant storage for engineering data, while Spark offers fast and in-memory data processing capabilities.
3. Internet of Things (IoT) and Sensor Data Management: With the proliferation of IoT devices and sensors in engineering projects, managing sensor data has become crucial. IoT platforms such as AWS IoT, Azure IoT Suite, and Google Cloud IoT provide tools and services to manage sensor data streams, perform real-time analytics, and integrate sensor data with other engineering data sources. These platforms enable organizations to harness the power of sensor data for monitoring, optimization, and predictive maintenance.
Incorporating these tools and technologies into Engineering Data Management processes can significantly enhance data management capabilities, improve data integration and analysis, and enable organizations to make data-driven decisions. However, it is essential to carefully evaluate the specific needs and requirements of the organization and select the tools and technologies that best align with those needs.
Best Practices for Effective Engineering Data Management
To ensure the successful implementation of Engineering Data Management, organizations need to adopt best practices that optimize data management processes, enhance data quality and consistency, enable collaboration and knowledge sharing, enforce security and data privacy measures, and establish robust backup and disaster recovery strategies. In this section, we will explore these best practices in detail.
Establishing a Data Management Strategy
A well-defined data management strategy is the foundation of effective Engineering Data Management. Organizations should establish clear goals and objectives for managing engineering data and align them with their overall business objectives. This strategy should outline the key components of the data management process, including governance, acquisition and capture, storage and organization, integration and analysis, and maintenance and lifecycle management.
Creating a data governance team comprising stakeholders from different departments and disciplines is crucial. This team can drive the implementation of data management policies, standards, and processes. They can also ensure that data management practices are aligned with industry best practices and regulatory requirements.
Developing clear workflows and processes for data management activities, such as data acquisition, storage, analysis, and maintenance, is essential. These workflows should be documented and communicated to all relevant stakeholders. Regularly reviewing and updating the data management strategy ensures that it remains aligned with evolving business needs and technology advancements.
Ensuring Data Quality and Consistency
Data quality and consistency are vital for effective Engineering Data Management. Organizations must establish processes and mechanisms to ensure that the data acquired and stored is accurate, complete, and reliable. Some best practices for ensuring data quality and consistency include:
1. Data Validation and Verification Techniques: Implementing data validation and verification techniques ensures that the acquired data meets predefined quality standards. Techniques such as data profiling, data cleansing, and data integrity checks help identify and rectify inconsistencies, errors, and duplicates in the data.
2. Data Standardization and Cleansing: Standardizing data formats, units, and naming conventions across different data sources improves data consistency. Data cleansing techniques, such as removing irrelevant or duplicate data, enhance data quality. Regularly auditing and cleansing data ensures that it remains accurate and up-to-date.
3. Data Auditing and Continuous Improvement: Conducting regular data audits helps identify data quality issues and areas for improvement. By analyzing data usage patterns and user feedback, organizations can continuously improve data quality and address any data management bottlenecks or challenges.
Enabling Collaboration and Knowledge Sharing
Effective Engineering Data Management requires seamless collaboration and knowledge sharing among stakeholders. Organizations should implement tools and platforms that facilitate collaboration, enable efficient communication, and promote knowledge sharing. Some best practices for enabling collaboration and knowledge sharing include:
1. Implementing Collaborative Tools and Platforms: Utilize collaborative tools such as project management software, document sharing platforms, and instant messaging applications to foster collaboration among team members. These tools allow for real-time communication, file sharing, and version control, enabling teams to work together efficiently.
2. Establishing Data Sharing Protocols: Define data sharing protocols to ensure that the right data is shared with the right stakeholders at the right time. Implement access controls and permissions to safeguard sensitive data while enabling authorized users to access and collaborate on shared data.
3. Encouraging Cross-Functional Collaboration: Foster a culture of collaboration and knowledge sharing across different departments and disciplines. Encourage engineers, designers, and other stakeholders to share their expertise, insights, and lessons learned. Establish cross-functional teams to address complex engineering challenges and promote innovation.
Security and Data Privacy Measures
Ensuring the security and privacy of engineering data is paramount. Organizations should implement robust security measures to protect sensitive information from unauthorized access, data breaches, and cyber threats. Some best practices for security and data privacy include:
1. Implementing Access Control and User Permissions: Define access control policies and user permissions to restrict access to engineering data based on roles and responsibilities. Implement multi-factor authentication and strong password policies to enhance security.
2. Data Encryption and Secure Transmission: Utilize encryption techniques to protect data both at rest and during transmission. Secure data transmission protocols such as Secure File Transfer Protocol (SFTP) or Virtual Private Networks (VPNs) can be employed to ensure secure data exchange between systems and stakeholders.
3. Compliance with Data Privacy Regulations: Stay updated with data privacy regulations, such as the General Data Protection Regulation (GDPR) or industry-specific regulations. Ensure that data management practices are compliant with these regulations, including obtaining consent for data collection, storage, and processing, and providing mechanisms for data subjects to exercise their rights.
Regular Data Backup and Disaster Recovery Planning
Data backup and disaster recovery planning are essential components of effective Engineering Data Management. Organizations should establish strategies and processes to ensure regular data backups and the ability to recover data in the event of data loss or system failures. Some best practices for data backup and disaster recovery include:
1. Backup Strategies and Redundancy Options: Implement backup strategies that meet the organization's recovery time objectives (RTO) and recovery point objectives (RPO). Regularly schedule automated backups of engineering data to multiple locations or storage mediums for redundancy and fault tolerance.
2. Testing and Updating Disaster Recovery Plans: Regularly test and validate the effectiveness of disaster recovery plans. Conduct drills to simulate data loss scenarios and ensure that recovery procedures are well-documented and up-to-date. Update disaster recovery plans as the organization's infrastructure and data management practices evolve.
3. Ensuring Business Continuity: Develop strategies to ensure business continuity in the event of data loss or system failures. This may include having redundant systems, backup power supplies, and failover mechanisms to minimize downtime and maintain operations.
By following these best practices, organizations can optimize their Engineering Data Management processes, enhance data quality and consistency, foster collaboration and knowledge sharing, enforce security and data privacy measures, and ensure business continuity in the face of data loss or system failures. These practices set the foundation for efficient and effective management of engineering data throughout its lifecycle.
Future Trends and Considerations in Engineering Data Management
Engineering Data Management is a dynamic field that continues to evolve as technology advances and organizations strive to harness the full potential of their engineering data. In this section, we will explore some future trends and considerations that will shape the landscape of Engineering Data Management in the years to come.
Artificial Intelligence and Machine Learning
Artificial Intelligence (AI) and Machine Learning (ML) have the potential to revolutionize Engineering Data Management. These technologies can automate data processing, analysis, and decision-making, enabling organizations to derive insights and make predictions from their engineering data at an unprecedented scale and speed. AI and ML algorithms can identify patterns, anomalies, and correlations in large datasets, empowering engineers and decision-makers to optimize processes, identify risks, and drive innovation.
By leveraging AI and ML, organizations can automate data quality control processes, detect data anomalies in real-time, and predict equipment failures or maintenance needs. These technologies can also enhance data integration by automating the mapping and transformation of data from various sources. As AI and ML continue to advance, they will become indispensable tools for effective Engineering Data Management.
Augmented Reality and Virtual Reality
Augmented Reality (AR) and Virtual Reality (VR) technologies are gaining momentum in the engineering field. They have the potential to transform how engineers interact with and visualize engineering data. AR and VR can provide immersive experiences, allowing engineers to visualize 3D models, simulate real-world scenarios, and identify design flaws or optimization opportunities.
With AR, engineers can overlay digital information onto the physical environment, enabling them to view engineering data in the context of the real world. VR, on the other hand, creates a virtual environment that allows engineers to explore and interact with engineering data in a more intuitive and immersive manner.
These technologies have significant implications for Engineering Data Management. AR and VR can facilitate collaboration among design teams, enable virtual design reviews, and improve communication and understanding of complex engineering concepts. As AR and VR become more accessible and affordable, their integration with Engineering Data Management will become more prevalent, enhancing the visualization and analysis of engineering data.
Internet of Things and Sensor Data Management
The proliferation of Internet of Things (IoT) devices and sensors presents both opportunities and challenges in Engineering Data Management. As more devices and sensors are connected, the volume of engineering data being generated increases exponentially. Organizations must develop strategies for efficiently managing and analyzing this vast amount of sensor data.
Sensor Data Management platforms and technologies will play a crucial role in handling IoT and sensor data. These platforms enable organizations to collect, store, analyze, and derive insights from sensor data streams in real-time. By integrating sensor data with other engineering data sources, organizations can gain a holistic view of their operations and make data-driven decisions.
As IoT and sensor technologies advance, we can expect to see increased integration with Engineering Data Management systems. This integration will enable organizations to leverage real-time sensor data for predictive maintenance, performance optimization, and resource allocation.
Ethical Considerations and Data Governance
As the importance of engineering data grows, ethical considerations and data governance will become even more critical. Organizations must ensure that they handle engineering data in an ethical and responsible manner, respecting privacy rights, security requirements, and regulatory compliance.
Data governance frameworks will need to evolve to address emerging challenges and changes in data privacy regulations. Organizations must establish clear guidelines for data collection, storage, access, and sharing to protect the privacy and confidentiality of engineering data. They should also establish mechanisms for data subjects to exercise their rights, such as the right to access, rectify, and delete their personal data.
In addition, organizations must consider the ethical implications of using engineering data, especially when leveraging AI and ML technologies. Transparency, fairness, and accountability in algorithmic decision-making are crucial to ensure that engineering data is used in a responsible and unbiased manner.
Continued Advancements in Data Storage and Processing
The ever-increasing volume and complexity of engineering data necessitate advancements in data storage and processing technologies. Organizations will require scalable and secure storage solutions to handle the growing amounts of data generated throughout the product lifecycle. Cloud storage platforms will continue to evolve, offering increased storage capacity, improved security features, and enhanced integration with Engineering Data Management systems.
Similarly, advancements in processing technologies such as in-memory computing, parallel processing, and edge computing will enable organizations to analyze and derive insights from massive datasets more efficiently. These advancements will reduce processing times, enable real-time analytics, and empower engineers and decision-makers with timely and accurate information.
In conclusion, the future of Engineering Data Management holds immense potential for organizations to leverage their engineering data for improved decision-making, innovation, and operational efficiency. With the integration of AI and ML, AR and VR, IoT and sensor technologies, ethical considerations, and advancements in data storage and processing, organizations can unlock new opportunities and gain a competitive edge in the rapidly evolving engineering landscape. By staying abreast of these trends and considering their implications, organizations can effectively navigate the future of Engineering Data Management and harness the full potential of their engineering data.
Conclusion
Engineering Data Management is a critical discipline that enables organizations to effectively acquire, store, organize, integrate, analyze, and maintain engineering data throughout its lifecycle. By implementing robust Engineering Data Management practices, organizations can unlock the full potential of their engineering data, drive innovation, improve decision-making, and gain a competitive edge in the industry.
Throughout this comprehensive guide, we have explored the definition and importance of Engineering Data Management, delved into the various components involved, discussed tools and technologies for effective data management, and explored best practices for implementation.
We have learned that data governance and policy, data acquisition and capture, data storage and organization, data integration and analysis, and data maintenance and lifecycle management are the key components of Engineering Data Management. By establishing clear data management strategies, ensuring data quality and consistency, enabling collaboration and knowledge sharing, enforcing security and data privacy measures, and implementing robust backup and disaster recovery plans, organizations can optimize their data management processes and derive maximum value from their engineering data.
Furthermore, we have explored the tools and technologies available for Engineering Data Management, including data management software, data integration and analytics tools, and cloud computing and big data technologies. These tools provide the necessary infrastructure and capabilities to handle the complexity and volume of engineering data, enabling organizations to effectively manage and analyze their data.
As we look to the future, we anticipate that Engineering Data Management will continue to evolve alongside advancements in technologies such as artificial intelligence and machine learning, augmented reality and virtual reality, internet of things and sensor data management, and data storage and processing. Organizations must also consider ethical considerations and data governance to ensure responsible and ethical use of engineering data.
In conclusion, Engineering Data Management is a vital discipline that empowers organizations to unlock the full potential of their engineering data. By implementing effective data management practices, organizations can enhance collaboration, improve decision-making, accelerate innovation, and achieve operational excellence. It is imperative for organizations to prioritize Engineering Data Management as they navigate the rapidly evolving landscape of engineering and data-driven industries.
Get our insights into what’s happening in business and the world of work; interesting news, trends, and perspectives from our Expert community, and access to our data & trend analysis.
Be first in line to read The 360˚ View by subscribing below.
Hire exceptional talent in under 48 hours with Expert360 - Australia & New Zealand's #1 Skilled Talent Network.